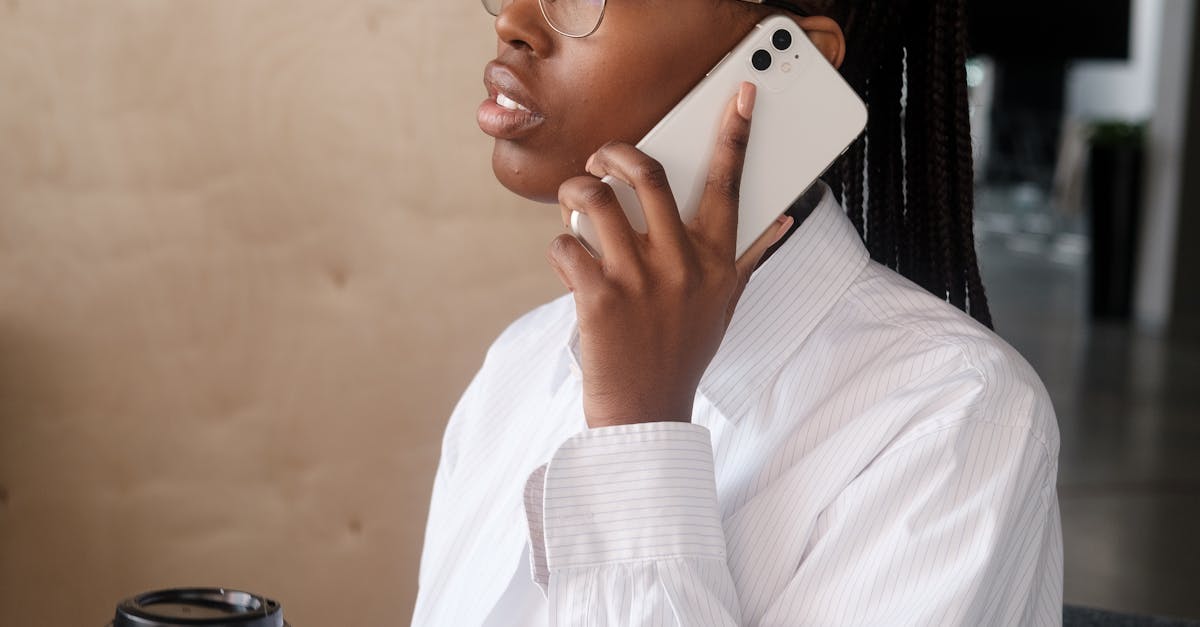
Table Of Contents
Inadequate Training and Knowledge Gaps
Many organisations invest heavily in advanced analytics tools but overlook a critical factor in their success: staff training. Employees may possess the best analytical software available, yet without adequate training, they often misuse these tools. This misuse not only leads to incorrect interpretations but also inhibits the overall effectiveness of analytics initiatives. Knowledge gaps in analytics and reporting can result in unreliable data outputs, which in turn affect decision-making processes.
To address this issue, organisations need to prioritise comprehensive training programs tailored to different levels of analytical competency. Investing in ongoing education will empower staff to effectively utilise analytics and reporting tools, fostering a culture of data-driven decision-making. By cultivating an understanding of basic analytics concepts and skills, companies can mitigate the risks associated with inadequate training and knowledge gaps, ultimately enhancing the value derived from their data initiatives.
Case Study
A prominent case study highlighting the misapplication of analytics involves a retail company that heavily invested in advanced analytical tools for its marketing department. The staff, while enthusiastic about utilising these new resources, lacked adequate training in data interpretation. This absence of foundational knowledge led to the misuse of the analytics and reporting platforms. As a result, campaigns based on flawed data analysis failed to resonate with the target audience, causing significant financial losses and decreased customer engagement.
Another notable instance of failure arose in a financial services firm that did not account for external variables when modelling client behaviour. This organisation relied on existing analytics and reporting systems without recognising shifts in the economic landscape, including emerging market trends and regulatory changes. When these external factors were overlooked, the firm made strategic decisions that ultimately harmed its competitive edge, demonstrating the vital importance of a holistic approach to data analysis in dynamic environments.
Ignoring External Variables
Ignoring external variables can lead to significant misinterpretations when organisations rely solely on internal data for decision-making. In many cases, analytics is constructed around limited datasets, often resulting in a narrow view of the market landscape. External factors such as economic changes, competitor actions, and social trends can greatly influence the success of a strategy. Without factoring in these variables, organisations risk making decisions based on incomplete information, diminishing the effectiveness of their analytics.
The importance of comprehensive analytics and reporting becomes particularly evident in industries affected by rapid market changes. Failing to incorporate external data into the analytics framework may result in missed opportunities or misguided strategies. For instance, a retail company might focus on internal sales figures while neglecting shifts in consumer behaviour or emerging competitors, undermining their overall business agility. Integrating external variables into analytics ensures a more accurate representation of the market, allowing for more informed and strategic decision-making.
Case Study
A prominent case study highlighting the consequences of inadequate training in analytics is that of a mid-sized retail company. Despite investing heavily in advanced analytics software, the staff struggled to utilise the tools effectively. Employees lacked the necessary training to interpret the data accurately. This resulted in misinterpretations that led to misguided marketing strategies. The failure to establish comprehensive training programs left significant knowledge gaps within the workforce, undermining the potential benefits of Analytics and Reporting.
In another instance, a financial services firm faced severe setbacks due to ignoring external variables that should have informed their analytics approach. The organisation relied on historical data without accounting for significant market changes such as regulatory shifts and evolving economic conditions. This oversight caused them to develop strategies based on outdated information. Despite possessing robust Analytics and Reporting capabilities, the inability to adapt the models to incorporate current and relevant external data led to poor decision-making and ultimately financial losses.
Failure to Iterate on Model Predictions
Analytics and Reporting are crucial components for organisations aiming to remain competitive in rapidly changing markets. However, many companies fall into the trap of relying heavily on initial model predictions without revisiting or updating them. This stagnation can lead to a misalignment with the current state of the business or market, resulting in ineffective strategies that do not resonate with the latest data. When models remain static, they fail to account for shifts in customer behaviour, competitive dynamics, or economic conditions, ultimately hindering decision-making processes.
A classic example of this failure can be seen in businesses that developed predictive analytics models based on historical trends. As these organisations neglected to revise their models periodically, they operated under outdated assumptions. Consequently, new opportunities emerged that went unrecognised, while existing risks were underestimated. By failing to iterate on model predictions, these companies not only missed chances for growth but also increased their vulnerability to competitors who were more agile and responsive to real-time insights derived from effective Analytics and Reporting practices.
Case Study
In a notable instance, a retail company implemented a new analytics platform aimed at enhancing decision-making processes. The team lacked adequate training in using the sophisticated tools provided by the system. This gap led to employees misinterpreting data and generating misleading reports. Consequently, the organisation faced difficulties in adjusting its promotional strategies, resulting in lost sales opportunities. The lack of proficiency in Analytics and Reporting ultimately hindered the potential benefits that the new system could have offered.
Another example involved a financial institution that relied on outdated predictive models for customer behaviour analysis. This institution failed to account for significant shifts in market dynamics such as economic downturns and changing consumer preferences. As a result, the institution continued to implement strategies based on stale models, leading to detrimental business decisions. Ignoring these external variables in their Analytics and Reporting compounded the challenges they faced, illustrating the necessity for continuous evaluation and adaptation in analytical practices.
FAQS
What are some common reasons for analytics failures in organisations?
Common reasons for analytics failures include inadequate training and knowledge gaps among staff, ignoring external variables that affect data interpretation, and failing to iterate on model predictions to keep them relevant.
How can inadequate training impact analytics outcomes?
Inadequate training can lead to staff misusing analytical tools, misinterpreting data, and ultimately making poor business decisions. This gap in knowledge can prevent teams from fully leveraging the capabilities of analytics.
Why is it important to consider external variables in analytics?
Ignoring external variables can result in misleading conclusions, as market changes and other factors may significantly influence data trends. Incorporating these variables helps create more accurate and actionable insights.
What does it mean to iterate on model predictions?
Iterating on model predictions involves regularly updating and refining analytical models based on new data and changing conditions. This process ensures that the insights generated remain relevant and effective for decision-making.
What lessons can be learned from case studies of analytics failures?
Lessons from these case studies highlight the importance of proper training, the need to consider external factors, and the necessity of regularly updating analytical models to avoid stale data leading to ineffective strategies.