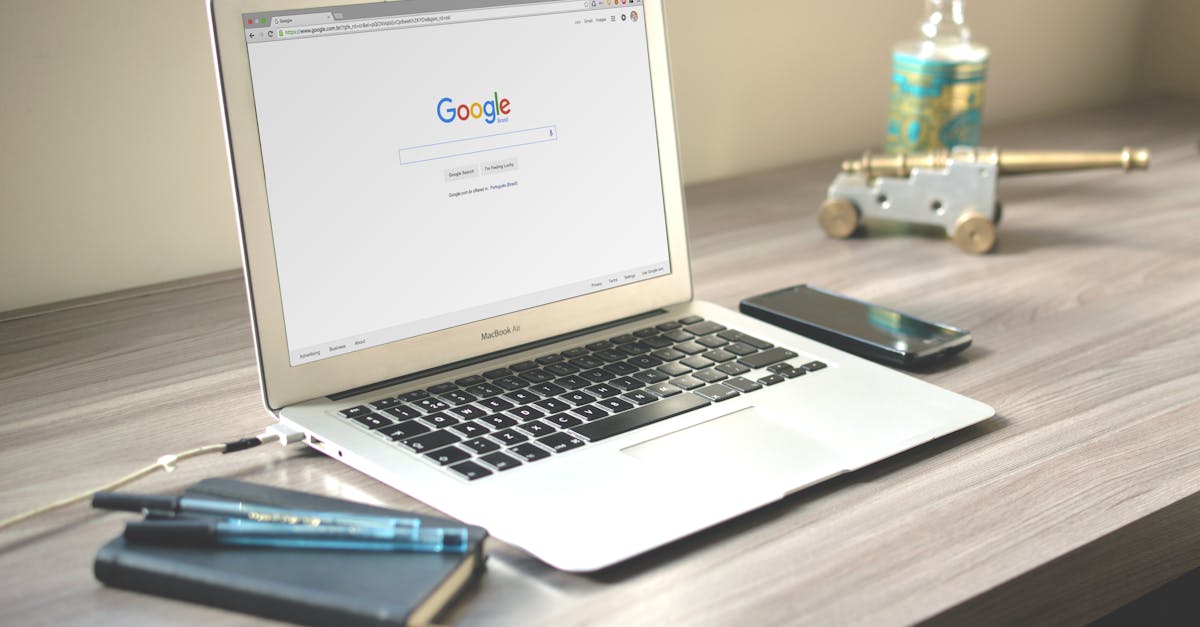
Table Of Contents
Analytics and Reporting Tools
Analytics and Reporting tools are essential in transforming raw data into meaningful insights. These tools enable businesses to collect, analyse, and visualise data effectively. Features such as automated reporting and custom dashboards provide users with the capability to monitor key performance indicators in real-time. By leveraging these technologies, organisations can improve decision-making processes and enhance overall operational efficiency.
The landscape of Analytics and Reporting tools is diverse, with options ranging from user-friendly platforms to more advanced solutions. Popular tools available in the market include Google Analytics, Tableau, and Microsoft Power BI. Each of these tools offers unique features tailored to different analytical needs, catering to both small businesses and large enterprises. The right choice hinges on the specific goals of the organisation and the complexity of the data at hand.
Popular Tools Used in the Industry
Numerous tools dominate the analytics and reporting landscape, catering to diverse needs within organisations. Google Analytics remains a staple for many, offering insights into website performance while tracking user behaviour. Tableau stands out in the realm of data visualisation, allowing users to create interactive dashboards that provide a detailed view of metrics. These tools help businesses extract meaningful insights from their data, guiding strategic decisions.
Another popular option is Microsoft Power BI, which integrates seamlessly with other Microsoft products to deliver robust analytics and reporting capabilities. This tool facilitates data manipulation and sharing, making it accessible for teams to collaborate. Similarly, QlikView provides a unique associative data model that enables users to explore data relationships easily. The combination of these tools enhances an organisation's ability to harness analytics and reporting effectively for improved outcomes.
Visualization Techniques
Visualisation techniques play a crucial role in transforming complex data into understandable formats. Effective visualisations help stakeholders grasp insights quickly and make informed decisions based on trends and patterns. Data can be showcased through various means, including charts, graphs, and dashboards. When utilising these techniques, it's essential to maintain clarity and simplicity to ensure that the information is accessible to all users.
In the realm of Analytics and Reporting, choosing the right visual representation can significantly enhance communication. Bar charts may be ideal for comparing quantities, while line graphs effectively illustrate changes over time. Incorporating colour codes and interactive elements can further enrich the user experience, making data more engaging. Ultimately, the goal is to present data in a manner that encourages exploration and deeper understanding, allowing users to derive actionable insights.
Best Practices for Data Visualization
Effective data visualisation plays a critical role in interpreting and communicating insights derived from Analytics and Reporting. Simplification is key; visuals should present data in a clear and concise manner. Avoid overwhelming audiences with excessive information or complex graphics. Employing a limited colour palette and consistent design elements can enhance clarity, making it easier for viewers to grasp the central messages without distractions.
Moreover, using appropriate chart types tailored to the nature of the data ensures accurate representation. For example, bar graphs work well for comparing quantities, while line charts are ideal for showcasing trends over time. Include informative labels and legends, facilitating immediate understanding. The best practices in data visualisation not only make the information more accessible but also improve the overall effectiveness of the Analytics and Reporting process.
Integrating Machine Learning
Integrating machine learning into analytics and reporting processes can significantly enhance data interpretation and decision-making. By leveraging algorithms that learn from historical data, organisations can identify patterns that may not be immediately visible. This capability allows businesses to optimise operations, improve customer experiences, and make more informed strategic choices based on predictive insights. As data volumes continue to grow, machine learning equips analysts with the tools needed to extract meaningful information efficiently.
The implementation of machine learning models requires a strategic approach to ensure they align with existing analytics and reporting frameworks. It is essential to have clean, well-structured data to train these models effectively. Collaboration between data scientists and business stakeholders is crucial for defining the objectives of machine learning initiatives. Creating a feedback loop helps refine models over time, improving their accuracy and relevance as organisational needs evolve.
Enhancing Insights Through Predictive Analytics
Predictive analytics has emerged as a transformative component of the analytics technology stack. By leveraging historical data and advanced statistical algorithms, organisations can forecast future trends and behaviours. This approach allows businesses to make informed decisions based on the insights gained from past performance, significantly enhancing their strategic planning processes. With effective analytics and reporting tools, teams can visualise these predictions, providing a clearer understanding of potential outcomes and enabling proactive strategies.
Integrating predictive analytics into everyday operations can lead to substantial improvements across various sectors. In retail, companies can use these insights to optimise inventory management and personalise customer experiences. In finance, predictive models assist in risk assessment and fraud detection. By harnessing the power of data through analytics and reporting, organisations can cultivate a data-driven culture, fostering innovation and driving competitive advantage in an increasingly complex marketplace.
FAQS
What is an analytics technology stack?
An analytics technology stack refers to the combination of tools and technologies used to collect, process, analyse, and visualise data for generating insights and making data-driven decisions.
What are some popular analytics and reporting tools used in the industry?
Some popular analytics and reporting tools include Google Analytics, Tableau, Microsoft Power BI, Looker, and SAS. Each tool offers unique features tailored for different analytics needs.
Why is data visualisation important in analytics?
Data visualisation is important because it helps to present complex data in a clear and understandable format, making it easier for stakeholders to grasp insights and trends, ultimately aiding in better decision-making.
How can machine learning be integrated into the analytics stack?
Machine learning can be integrated into the analytics stack by incorporating algorithms that analyse historical data to identify patterns and make predictions. This can enhance the depth of insights derived from data.
What are some best practices for effective data visualisation?
Best practices for effective data visualisation include keeping visuals simple and uncluttered, choosing the right type of chart for your data, ensuring that visuals are accessible to all users, and providing clear labels and legends for better understanding.