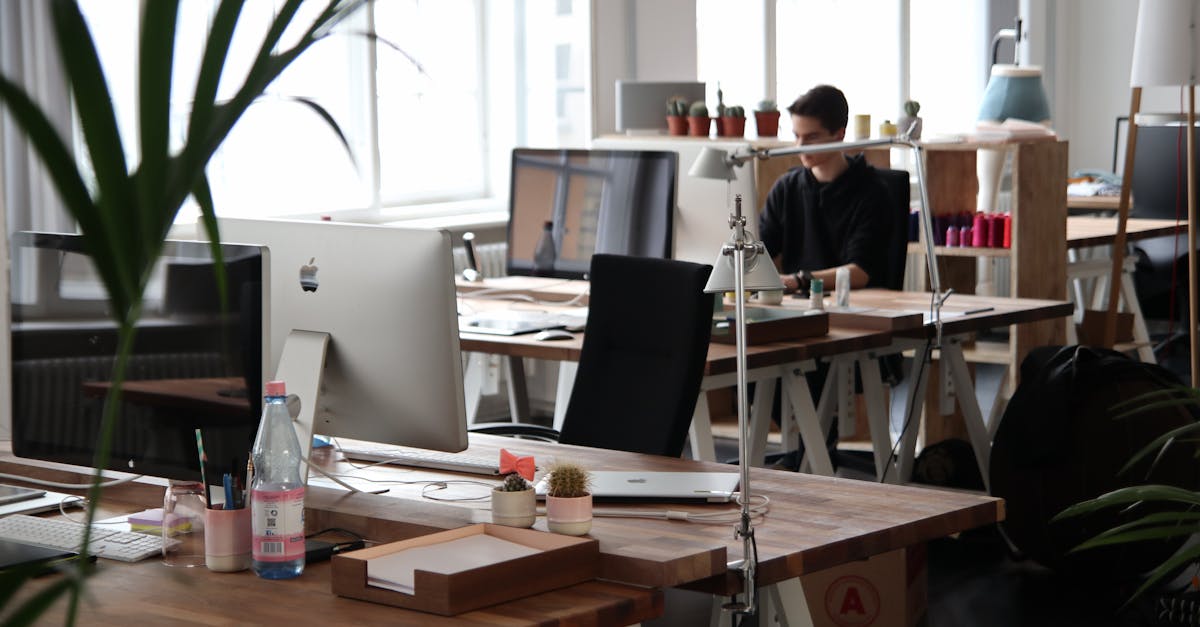
Table Of Contents
Machine Learning and Big Data Integration
The integration of machine learning with big data technologies represents a significant advancement in the field of analytics and reporting. By harnessing algorithms that can learn from vast datasets, organisations can uncover patterns and insights that would be nearly impossible to identify using traditional analytical methods. Machine learning models can process diverse data types, allowing businesses to make more informed decisions based on predictive analytics and improve operational efficiencies.
Furthermore, this integration enhances the capacity to analyse real-time data. As machine learning systems continuously evolve, they refine their predictive capabilities, thereby providing organisations with timely insights. This dynamic capability enables businesses to adapt quickly to market changes and customer behaviours. Effective analytics and reporting tools are essential, facilitating the interpretation of complex datasets and supporting data-driven decision-making processes across various sectors.
Enhancing Predictive Analysis Using ML
Machine learning (ML) significantly enhances predictive analysis by processing vast datasets far more efficiently than traditional methods. This advanced capability allows organisations to uncover complex patterns and trends in their data, providing deeper insights for informed decision-making. By leveraging techniques such as regression analysis, classification algorithms, and clustering, businesses can generate forecasts that are not only accurate but also relevant to their operational context. This synergy between ML and big data fosters a more robust approach to analytics and reporting, ultimately leading to better business outcomes.
Incorporating ML into predictive frameworks allows for continuous learning and adaptation, ensuring that models remain relevant as new data flows in. This proactive approach to data analysis means businesses can quickly identify shifts in consumer behaviour or market trends, allowing them to adapt strategies accordingly. Additionally, the automation of analytics and reporting processes reduces the burden on data analysts, enabling them to focus on interpretation rather than data manipulation. This evolution in analytics enhances the overall agility of organisations, positioning them to respond effectively in a rapidly changing business landscape.
Cloud Solutions for Big Data Analytics
Cloud solutions have revolutionised the way organisations approach big data analytics. Through scalable infrastructure, businesses can process vast amounts of data without the need for substantial upfront investment in physical hardware. This flexibility allows companies to adjust resources according to their current needs, which is particularly beneficial during peak times when data influx is high. Many cloud services offer advanced tools that facilitate real-time analytics and reporting, enabling teams to derive insights promptly and make informed decisions.
Evaluating cloud providers is crucial for maximising the effectiveness of big data analytics. Each major provider, including AWS, Azure, and Google Cloud, offers unique features tailored to different organisational needs. Factors such as cost, scalability, and the availability of specific data analytics tools can significantly influence the decision. Integrating cloud solutions with existing data strategies can enhance overall efficiency and improve the capability for comprehensive analytics and reporting.
Evaluating AWS, Azure, and Google Cloud
AWS, Azure, and Google Cloud are leading platforms for big data solutions, each offering distinct features that cater to various analytics needs. AWS provides a robust suite of tools for data storage, analytics, and machine learning, making it a popular choice for businesses aiming to harness large datasets. Its integration with services like Amazon Redshift and AWS Glue enhances the capabilities for real-time analysis. Azure, meanwhile, excels in its seamless integration with Microsoft products, allowing for effective data management and streamlined analytics workflows. The platform supports various programming languages and frameworks, making it user-friendly for organisations accustomed to a Microsoft environment.
Google Cloud stands out with its strengths in artificial intelligence and machine learning, particularly through BigQuery and Dataflow. These tools enable efficient handling of data analytics and reporting tasks, ensuring that users can derive insights quickly from their datasets. Additionally, Google Cloud emphasises collaboration and accessibility, allowing teams to work together on analytics projects regardless of location. Each of these cloud service providers has unique capabilities, and the choice ultimately depends on specific business requirements and existing infrastructure.
The Importance of Data Security
Data security is paramount in the realm of big data. As organisations harness vast amounts of information for analytics and reporting, the risks associated with data breaches and cyberattacks grow significantly. Maintaining the integrity of sensitive data involves implementing robust security measures, including encryption, access controls, and regular audits. These strategies not only safeguard information but also protect the reputation of the organisation against potential fallout from data mishandling.
Incorporating data security protocols into analytics and reporting processes is essential for compliance with regulations and standards. Governments and institutions worldwide continue to enact stringent laws regarding data protection. Organisations must remain vigilant in ensuring that their practices align with these requirements. By prioritising data security, businesses enhance their overall operational resilience while fostering trust among consumers who increasingly value transparency regarding how their information is managed.
Protecting Sensitive Information in Big Data
Data security remains a critical concern in big data environments, particularly when handling sensitive information. Sophisticated data breaches can expose personal details, financial records, and proprietary business information, leading to severe repercussions for both individuals and organisations. Implementing robust encryption methods protects data at rest and in transit, ensuring that even if unauthorized access occurs, the information remains unreadable. Additionally, regular audits and compliance with international privacy standards, like GDPR and Australia's Privacy Act, should form a key aspect of any data management strategy.
In the realm of Analytics and Reporting, safeguarding sensitive data requires a multi-faceted approach. Access controls must be meticulously established, limiting data visibility according to user roles and responsibilities. Furthermore, leveraging advanced analytics tools equipped with anomaly detection can help identify suspicious activities in real time. Cultivating an organisational culture that emphasises data stewardship and security awareness will further strengthen the protective measures essential to mitigating risks associated with big data analytics.
FAQS
What is the role of machine learning in big data analytics?
Machine learning plays a crucial role in big data analytics by enabling algorithms to analyse vast datasets, identify patterns, and make predictions, enhancing the overall accuracy and efficiency of predictive analysis.
How can cloud solutions benefit big data analytics?
Cloud solutions offer scalability, flexibility, and cost-effectiveness for big data analytics, allowing businesses to efficiently store, process, and analyse large volumes of data without the need for extensive on-premises infrastructure.
What factors should be considered when evaluating cloud platforms for big data?
Key factors to consider include pricing models, data storage options, processing capabilities, ease of integration, security features, and support for machine learning frameworks when evaluating platforms like AWS, Azure, and Google Cloud.
Why is data security important in big data analytics?
Data security is essential in big data analytics to protect sensitive information from breaches and unauthorized access, ensuring compliance with regulations and maintaining customer trust.
What measures can organisations take to safeguard sensitive data in big data environments?
Organisations can implement encryption, access controls, regular audits, and security protocols to safeguard sensitive data in big data environments, helping to mitigate risks associated with data exposure and loss.